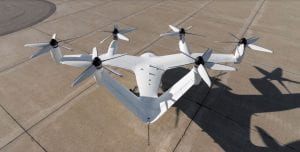
Demand Modeling for Air Taxi Service
Excitement is growing within the aviation community as improvements in battery technologies offer the potential for dramatically lower operating costs for new classes of electric propulsion aircraft. By reducing aircraft operating costs by as much as 30%, electric propulsion could transform both air and surface transportation [1]. The term “on-demand mobility” (ODM) has been interpreted in an aviation context as an air service between origin-destination pairs located at dispersed…
Read more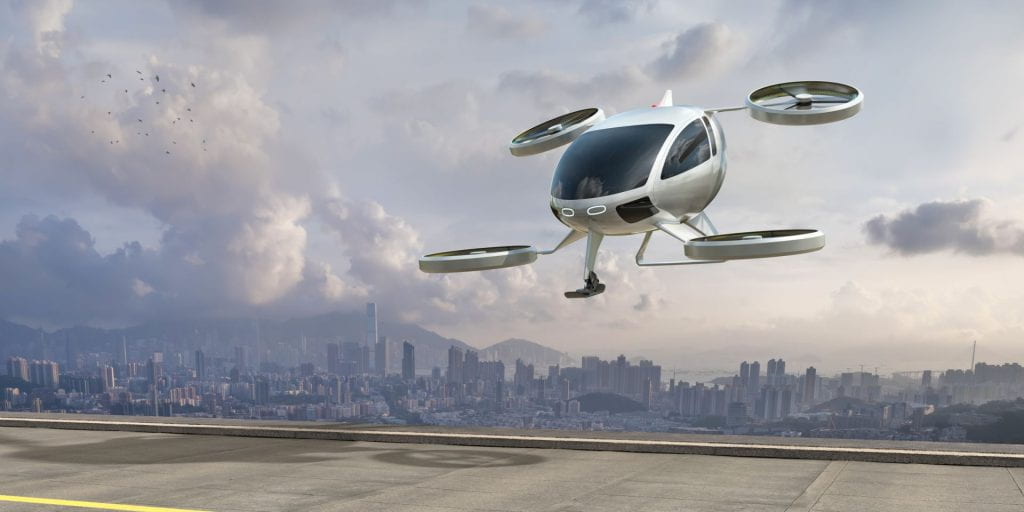
Commuter Air Taxi Demand in 40 US Cities
Advancements in electric propulsion and autonomy technologies are leading to the development of a new class of electric vertical takeoff and landing (eVTOL) aircraft. These aircraft may complement existing transportation systems by providing on-demand or scheduled air taxi service within urban areas. Through their ability to fly over congested ground transportation, air taxis may offer door-to-door travel-time savings for commute trips…
Read more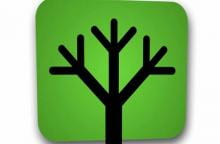
LARCH: A Freeware Package for Estimating Disrete Choice Models
LARCH is a software package written in Python and C++ by Jeffrey Newman that can be used to estimate multinomial, nested, and cross-nested logit models. The software exploits computational opportunities that arise from the use of semi-aggregate data (where the explanatory data for choice scenarios are not necessarily unique for each decision-maker) in discrete choice models. This data feature is commonly encountered with large transactional databases that have limited consumer information…
Read more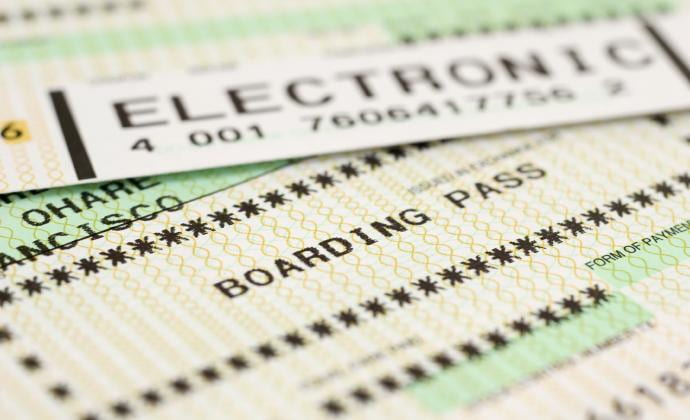
Revenue Management for NDC
The airline industry is investing in new technologies such as New Distribution Capability (NDC) technologies that more easily display offers that are tailored to individual customer preferences. As part of the research portfolio of the ATL@GT Center that Dr. Garrow directs, we are developing a competitive RM simulator that…
Read more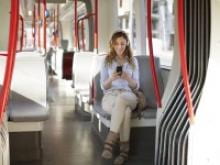
National Transit Database
The National Transit Database (NTD) is the primary source for information and statistics on the transit systems of the United States. The State of Georgia and more specifically, the Georgia Department of Transportation (GDOT) as a recipient of funds from the Federal Transit Administration (FTA) is required to report service and financial data for all rural transit systems in Georgia that receive Section 5311 funds on an annual basis. As part of this project, Georgia Tech researchers work…
Read more